Pass Amazon AWS Certified Machine Learning - Specialty Exam in First Attempt Easily
Latest Amazon AWS Certified Machine Learning - Specialty Practice Test Questions, Exam Dumps
Accurate & Verified Answers As Experienced in the Actual Test!
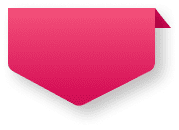
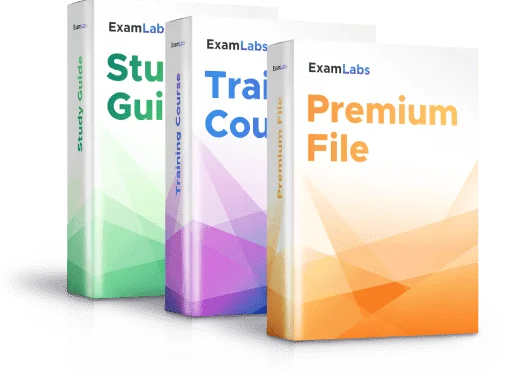
Check our Last Week Results!



- Premium File 369 Questions & Answers
Last Update: Mar 13, 2025 - Training Course 106 Lectures
- Study Guide 275 Pages
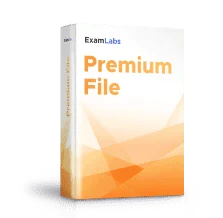
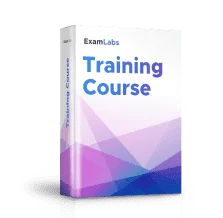
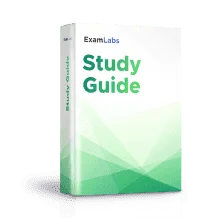
Download Free Amazon AWS Certified Machine Learning - Specialty Exam Dumps, Practice Test
File Name | Size | Downloads | |
---|---|---|---|
amazon |
1.6 MB | 1128 | Download |
amazon |
1 MB | 1232 | Download |
amazon |
1.4 MB | 1237 | Download |
amazon |
1.5 MB | 1227 | Download |
amazon |
1.4 MB | 1373 | Download |
amazon |
902.9 KB | 1518 | Download |
amazon |
1.2 MB | 1673 | Download |
amazon |
866.6 KB | 1725 | Download |
amazon |
610.6 KB | 1826 | Download |
amazon |
231.7 KB | 1918 | Download |
amazon |
856.9 KB | 1968 | Download |
amazon |
606.8 KB | 2086 | Download |
amazon |
566.9 KB | 2093 | Download |
Free VCE files for Amazon AWS Certified Machine Learning - Specialty certification practice test questions and answers, exam dumps are uploaded by real users who have taken the exam recently. Download the latest AWS Certified Machine Learning - Specialty AWS Certified Machine Learning - Specialty (MLS-C01) certification exam practice test questions and answers and sign up for free on Exam-Labs.
Amazon AWS Certified Machine Learning - Specialty Practice Test Questions, Amazon AWS Certified Machine Learning - Specialty Exam dumps
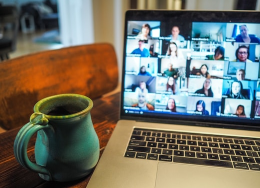
Introduction
1. Course Introduction: What to Expect
Let's begin by setting some expectations. The AWS Certified Machine Learning Specialty exam isn't like any other AWS exam. If you've taken other AWS certifications, you probably expect to be tested on extremely deep knowledge of AWS services and how to architect systems composed of those services to solve specific problems. When it comes to the AWS Sage Maker service, much of that is still true on the machine learning exam. You'll need a lot of depth on Sage Maker, how it integrates with other systems, how to secure it, and low-level details on how its many built-in algorithms work. But what's weird about this exam is that it's not all about AWS. About half of the exam isn't about AWS at all. It tests your general machine learning knowledge. How do deep neural networks work? What sorts of algorithms should you choose for specific problems? How do I tune those algorithms to achieve the best results? A lot of what this exam tests you on has nothing to do with AWS specifically at all. What's also unusual about this exam is that it seems designed to confound people who don't actually have years of industry experience in machine learning. A lot of what it tests you on are the nuances of tuning your models, identifying and preventing overfitting, achieving consistent results, and scaling models up to massive data sets. These aren't things that are generally covered in introductory machine learning courses. These are things that are usually learned through experience. This course will attempt to change that and cover those nuances of regularisation and hyperparameter tuning that are often ignored in other machine-learning courses. I've heard stories of well recognised AWS experts walking out of this exam asking themselves, what the heck was that? You can't rely on your AWS expertise to pass this exam. You also need to be a genuine machine learning expert. We're going to do our best to get you there, but be prepared. This is a very difficult certification to achieve. Here's what you can expect as you go through this course. The AWS Certified Machine Learning Exam is broken up into four domains, and those will match the four main sections in this course. The first domain is data engineering, which makes up 20% of the questions on the exam. And this is how the AWS Exam Guide describes what they're looking for. But we'll go into specifics about our storage solutions, including S3, three data lakes, and DynamoDB. We'll talk about transformations using glue and glue ETL. We'll talk about how to stream data using Kinesis, and specifically Kinesis video streams, which pair nicely with Amazon's recognition service. And we'll talk about some workflow management tools such as data pipelines, AWS batch, and step functions. The next domain is exploratory data analysis, and that counts for 24% of the exam questions. In this section, we'll dive into some data science basics, including what Scikit-Learn is all about, what some basic data distributions are that you might see, and some basic concepts like trends and seasonality. Then we'll go deeper into the analysis tools that AWS offers, including Athena, QuickSite, and the Elastic MapReduce service. We will also spend some time on Apache Spark. Even though that's not technically an AWS product, it is heavily used in the data analysis world, and you will be tested on it. We'll also talk about the world of feature engineering. This isn't talked very often. How do I impute missing data? How do I deal with outliers? How do I manipulate data to categorise it? How do I transform my data to fit into the algorithms that I'm working with? What's one-hot encoding all about? And how do I scale and normalise my data before feeding it in to train a machine learning model? Next we'll go into the world of modelling, which is the biggest section. This accounts for 36% of the exam questions, and it's extremely important. This is where we go into some more general deep learning stuff. So if you're not familiar with the world of deep learning yet, we'll give you a crash course, and you'll learn all about multilayer perceptrons, convolutional neural networks, and recurrent neural networks. And we'll spend a lot of time on how to tune those networks and the regularisation techniques you can use to get the best performance out of a neural network by getting just the right parameters on them and the right topology. We'll also spend a lot of time on Sage Maker. Sage Maker is Amazon's flagship service for the world of machine learning. We'll cover its architecture and the long list of its built-in algorithms that you see here. I got to know what each one of them does and how they work. We'll talk about its automatic model tuning capabilities, dive into an example of that, and talk about how Sage Maker can integrate with other systems, including Apache Spark. We'll also talk about Amazon's higher-level AI services that are at a higher level than Sage Maker. Understand, translate, poly, transcribe, lex, and recognise are among them. We'll also touch on some of the newer services, such as personalised forecasts and textTRACK. Although they don't seem to be on the exam yet, I think it's a matter of time before they show up, and we'll talk a little bit about their deep lens product as well. We'll also dive into evaluating and tuning machine learning models. You need to know what a confusion matrix is, you need to know what RMSC is, and you need to compute precision and recall and F1 scores. And you need to have a deep knowledge of what ROC and AUC are all about. You will by the time you're done with that section. The last domain is machine learning, implementation, and operations. And this is primarily on the exam concerning Sage Maker operations. So we'll spend a lot of time on the guts of Sage Maker. Again here. How do I use containers within SageMaker to provide my own training models? How do I deal with security under Sage Maker? How do I choose the right instance types for my algorithms? How can I do live A/B testing? How do I integrate with other systems, including TensorFlow? How can I push Sage Maker models out to the edge using Sage Maker, Neo, and Greengrass to actually run machine learning on edge devices? How do I take advantage of pipes to accelerate my training, and what's the deal with elastic inference and inference pipeline is all about? And once you're through all that, it's a lot to digest, but you'll be ready to go get certified at that point. It's time to go take some practise exams and sign up for the real thing. And hopefully, you too will get a certificate like this at the end of your journey. There's a lot to learn and a lot to cover, but we've done the best to focus on the specific topics this certification exam focuses on. If you can master the material we're about to present and pass the practise exam, you should be in good shape as you head into the real exam. Let's start this journey and get you included in the exclusive club of certified machine learning experts.
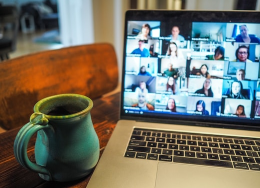
Data Engineering
1. Section Intro: Data Engineering
The first domain we'll cover is data engineering. You'll find that in practice, you spend a lot of time just collecting and processing the data you need to train your machine learning algorithms. Often, this is a larger effort than building the machine learning algorithms themselves. There's a lot of overlap with the AWS Certified Big Data Specialty Exam in this section, and if you already hold a Big Data certification, you'll find that this section is largely a review. In general, you'll find that the machine learning exam won't go into as much depth on these topics as the big data exam did, but you will be expected to know how to construct a data pipeline using services such as S3, Glue, Kinesis, and DynamoDB. The challenge is to get your data where it needs to be for training your models in a manner that can deal with massive scale and with strict security requirements. For this section, I'm handing things off to Stefan Merek, who is a recognised expert in AWS storage solutions and a certified AWS Solutions Architect, in addition to being a very popular instructor in the world of AWS certification. So take it away, Stefan, and walk us through the world of data engineering in AWS.
2. Amazon S3 – Overview
So, let's get started with S3. So first, you may already know what S3 is, but I just want to go over the quick points you need to know to pass the AWS Machine Learning Exam. So S3 allows you to store objects or files in buckets, and that will be the centre piece of all the stuff you will do in AWS US. The buckets must have a globally unique name, and the objects are files, and they have a key. And the key is the full path of that file. For example, you have the bucket, myfile.TXT, but you can also have a very, very long file name, as we'll see in this course. My folder, another folder, or myfire.txt are all possibilities. It turns out they're not actually folders—it's just a very long key name. It will be interesting when we look at partitioning. So we'll see how to do partitioning in the next two slides. And this will be extremely helpful when we have queries on Athena and when we start partitioning our data to better query it. So the max object size is five terabytes. So you have to remember that if you have a very large data set, then you cannot fit in only one object. It needs to be split into different objects because the max object size is five terabytes. And finally, we can add object tags that are key-value pairs, and they will be very helpful for classifying our data and for security and life cycle. So now for machine learning regarding Three, what do we have? What's going to be the backbone for many AWS ML services? For example, Sage Maker, as Frank will show you, will be helpful to create a dataset like this, which is why the whole purpose of this section on data engineering is to create datasets of infinite size. You don't have to provision anything for using S3. You have a durability of eleven and nine. So that means that your data is secure, and you shouldn't lose an object for a long time. And then there's this decoupling of storage, which is S3, that we'll see in a second, from everything that is compute based. So EC Two, Athena, Redshift, spectrum recognition, glue, all these things are on the compute side, and they're completely separate from the storage side, which is S3. And that paradigm allows you to create a massive data lake in S3 that is extremely scalable and that works with all the other compute services. It's a centralised architecture. So that means that all your data can be in one place, and there's object storage. So it means that you support any file format. S3 doesn't even look at your data. You just put whatever you want in there. So you can work in any kind of format. For example, CSV, Jason's Parquet, RC Avro, or protobuff. That makes S3 a perfect use case for a day in the cloud. So now let's talk about data partitioning. Data partitioning is a pattern for speeding up range queries. For example, if you use Amazon Athena, which is a service to query data in a serverless manner, and we'll see this in the hands-on, then you may want to partition by date. For example, we have three buckets, and then we have my data set, and then we'll have year, month, day, and hour, and these are called partitions because they're going to separate our data set into different folders and partitions. And then finally we have our data in CSV format, which will be very helpful if we start querying our data a lot by date because it will allow us to quickly find the right data sets based on the year, month, day, and hour that we choose. But if you want to query by product very often, maybe you want to reorganise your partitioning scheme so that my data set has a product ID first as the first partition and then your data is in it, which will allow us very quickly to find the right data for the defined product ID. So you can define whatever partitioning strategy you like. But the concept of date and product is a very common one, and some of the tools we'll use, for example, Kinesis, will partition the data for us. So now let's go ahead and create our first three buckets. So I'm going to Amazon S3, and in there I'm going to create a bucket. As you can see in my account, I already have many, many buckets. But I'm going to create a new bucket, and I'll call it AWS Machine Learning Stefan. And as you can see, the name has to be global and unique. So if you try to use that name, it won't work for you. I'll choose a region that's close to me, and then I'll click on Create, as we'll see some of the options that are important for the exam in the next section. So in my bucket, I'm going to create my first folder, and I'll call this Instructions because this is an instruction that I gave, and I'll click on Save. So this is where I'll place a little bit of data into my few buckets. Here, I'm going to create a partition scheme. And so I'm going to first put down the year 2019. Then I'm going to go one more down. I'm going to create a folder that represents the month. So I'll enter 10 and press the save button. Then I'll upload and click on the day. So I create a folder and the day is going to be 23 Save. And then I click one more upload and I'm going to choose a file and I'll choose the Instructor Data CSV and click on Upload. And here we go. So we have our first dataset uploaded to Amazon S3. And this is the file that you can download by just clicking on "Download," and you can visualise what it is. As you can see, this file is a very simple file. This is a CSV file that just has my name and Frank's name in it, the name of this course, and the love meter. Okay, so that's it. Just for very quick introduction to S3, we'll be doing, obviously, a lot more stuff with S3, but we have created a few directories. We've uploaded a file, we've done some partitioning, and now we're ready to move on to the next part. So see you in the next lecture.
3. Amazon S3 - Storage Tiers & Lifecycle Rules
So now let's talk about the storage tiers in S3. So, by default, when you upload data to S3, it is Amazon S3 standard general purpose. But there are ways for you to specify different storage tiers, and this will change the pricing, usually decreasing it based on the kind of restrictions you want to have. So there is S3 standard infrequent access for data that is infrequently accessed. There is S3 One zone IA, or infrequent access, for data that you're willing to lose because it can be in only one availability zone in AWS, which means only one data center, and Amazon S3 intelligent tiering, where Amazon will figure out where our data should be put to optimise the pricing. And Amazon glitch here for archives. Going into the exam, you only need to remember a few things: there is a standard for frequently accessed storage and a tier for infrequently accessed storage (IA 3 or S3 one zone IA). Then there is this intelligent tiering, and again, these archives. So going into the exam, the important thing is going to be standard and glacier. You don't need to remember any of these numbers; I just put them here for reference. Okay. But what you need to remember is that there are different tiers in S3, and each tier will cost a bit less if you go to the right. So standard cost a lot. And then if you go all the way to Glacier, then it costs very little to store data. And the important thing to remember is that the more you use the data, the more you want it to be in the Standard tier. And the less you use the data, the more you want it to be in the glacier tier. Okay, and so how do you move objects between tiers? Well, for this, there is a lifecycle rule. And so it's a set of rules to move data between different tiers in order to save storage costs. So for example, you're going to go from general purpose when you use the data very often to infrequent access. When you use the data, very little is likely to glitch. At that point, you know that you just need to archive the data and not use it at all. So this is called "transition actions," where objects will be transitioned to another storage class. So for example, you can say, "I want to move objects to S3 IA after 60 days after creation, and then move them to Glacier for archiving after six months, where we can have expiration actions, where we can delete objects on our behalf." So for example, we say, "Okay, these access logs and files, we don't want to have them after six months, and so you can delete them." So let's go ahead and see how we can create these lifecycle rules. Okay, so back in S-3, I can go right into my data set, so I'm going to go find it in here. And if I click on this, we can see on the right-hand side that the storage class is standard. So if I click on the properties here and I click on "Storage class," it is standard. But as we can see, there's tonnes of different storage class that we have all these right here and with a different kind of billable and what it is designed for. Okay, so if I wanted to change this to, for example, standard IA for infrequent access, I could just click and click on Save, and now my object is moved into a different storage class. So, likewise, for Glacier, I could just click here and click on Save to have this in Glacier. But I won't do it because I won't be able to use that object. So the important thing here is to remember that you can transition objects between storage classes directly from the UI, but we can also, as we said before, go back to our buckets. We can set up some properties and some management, excuse me. And then we can have lifecycle rules. So I click on Lifecycle and then add Lifecycle Rule, and I enter the rule for the folder name instructors. I believe it was called Instructors. So let's have a look. Yeah, instructors. So, instructors with an S, follow the Lifecycle Rule. And here I'm going to add a filter to limit scope to just this prefix. Okay, click on Next, and then we're going to say, okay, you want to have the current version and add a transition. And the transition is that you want to go to Standard IA after 30 days, and then you transition to Glacier after 60 days. And as you can see, transitioning small objects to Glitch here will increase costs. But that's fine, I'll delete this rule anyway, okay, click Next, and then we'll configure the expiration for the current version, and we'll say, "Okay, please delete the object after 425 days, click Next, and save." And we have defined our first lifecycle role. So I'm not going to wait 30 days and then 60 days to show you that things do move between tiers. But you can get the idea that the files in here will move into Standard IA after 30 days, glacier after 60days, and will expire after a year or so. OK, so that's it. All you need to know for this lecture, and I'll see you in the next one.
4. Amazon S3 Security
S3's security is last but not least. Let's start with three-factor authentication for objects. There are four methods for encrypting objects in S3. There is SSE S3, where we'll encrypt S3 objects using a key that is handled and managed by AWS SSE, Kms. We will want to use the AWS Key Management Service to manage encryption keys. So we have more control over the keys. Then it gives us additional security because now we can choose who has access to this KMS key. In addition, we get an audit trail for KMS key usage. Then we have SSEC when we want to manage our own encryption keys outside of AWS. And finally, client-side. Encryption. We want to encrypt the data outside of AWS before sending it to AWS. So SSE, by the way, stands for server-side side Encryption. That means that Alice will do the encryption for us, which is different from client side encryption, or CSE. So from a machine learning perspective, only SSE3 and Ssekms will most likely be used. So you don't need to really remember what SSEC and client-side options are for this exam. So I will just do a deep dive into SSE 3 and SSE Kilometers. So, here's what it looks like. We have the Amazon S3 buckets, and we want to put an object in them. So we're going to send that object into S3, and then the object will arrive in S3. And for SSE S3, S3 has its own manage data key, and it will take that key plus the object and perform some encryption, then add this to your bucket. So, as we can see here, this key is managed by S3. We have no idea what it is, we don't manage it, and that's fine. Then there's SSE km, which follows the same pattern in km. We have our object, and we want to insert it into S3. So we'll send it to SRE, and then the key used now for encrypting that object is generated thanks to the KMS Customer Master Key. And this KMS Customer Master Key is something that we can manage ourselves within AWS. By employing this pattern, we gain more control, possibly more safety, and possibly a greater sense of security. And so AWS will use this custom master key and a datakey to encrypt the object and put it into the bucket. So that's it. That's the difference here. As you can see here in SSEKMs, we have control over how the customer-managed master keys will be used. In the previous example, it was only 3 who had its own data key. So now, for S-three security, how do we manage access directly into S3? It could be user base, so we can create Im policy. and IAM is identity access management. That means we need to specify which APIs Auser should be able to use on our buckets. Or it can be resource-based, where we say, "Okay, we want to set an overall bucket policy, and there will be a bucket-wide rule from the SB console and it will allow cross-account access and so on" and define how the users can access objects. And we could also have ACL, which is an object access control list, which is finer grain, and bucket ACL, which is less common. So going into the exam, the really important thing is to understand user im policies and bucket policies. So let's have a look at bucket policies. They're JSON documents, and you can define the buckets and objects. You can define a set of APIs to allow or deny, for example, "put object" or "get object." And then the account principle is the user's account to apply the policy to. So we can use a three bucket policy for multiple things. number one, to grant public access to the buckets. Number two: to force objects to be encrypted at upload time or to grant access to another account. They are very common in AWS. Now there is a way to set default encryption for your buckets instead of using bucket policies. So the old way was to set a bucket policy and refuse any request that did not include the right header for encryption. So this is what it looked like before, but nowadays you can just use a new way, which is called "default encryption" in S3. And using default encryption, there will be a way to just say "S3" to encrypt every single object that is sent to it. So you need to know just that the bucket policies are evaluated before the default encryption. So if we go to S3 now and go to Overview and look again at our data points, we look at Instructor Data CSV and click on the properties. As you can see, there is currently no encryption, but I can click on it and select SSE S3, which is 256, or AWS kms. And we need to set up a key to anchor the data with. So we can either set up one managed by AWS or create our own or send our own custom key arm. So I'll just type SSEs3 in here and click Save. And as we can see now, this object's Instructor Data CSV is fully encrypted. Another way to do it is to click on the bucket, click on Properties, and go to Default Encryption. And here we can set a default encryption for all the objects in our buckets, but only for the new ones that are uploaded. So we'll say okay; we want AES 256. That means that we're going to say, "Okay, all the objects should be encrypted with SSEs free, click on Save, and we're good to go." So now if I go back to my overview and I go back to my data set, So I'm going to go back to my objects. I'm going to delete this one, okay? And I'm going to upload it all over again. So something we should see now is that the object itself is already encrypted with AES 256. So automatically, thanks to the default encryption, AES 256, we were able to encrypt that object. So another way of doing it would be to use bucket policies. So I'm not going to go into the details of bucket policies because you don't need to know them for the exam, but just know that we could set a bucket policy here to provide access to the files in our buckets, okay, to other users or AWS services. So finally, other things for security you need to absolutely remember going to the exam. The first one is networking for a VPC endpoint gateway. So when you use S3, right now, when I do it, I go over the public Internet, and all my data goes over the public Internet. But if I have private traffic within my VPC or virtual private cloud, then I want it to remain within this VPC. And so I can create what's called the VPC endpoint gateway for three And they will only allow the traffic to stay within the VPC instead of going through the public website for S3. To make sure that all the private services, for example, AWS, Sage Maker, as Frank will show, you can access S3. So Frank will be reiterating that point, obviously, when we go into Sage Maker. But you need to remember this very important fact for the Iris exam. If you want your traffic to remain within your VPC Four, S, and not go over the public web, then creating a VPC endpoint gateway is the way to go. There's also logging and auditing. So you can create S3 access logs to store in other S3 buckets to make sure that you can see who has made a request to your data. And all the API calls can belogged into something called Cloud Trail. Cloud Trail is a service that allows you to look at all the API calls when made within your account. And that could be really helpful in case something goes bad and you need to remember who did what and why. Finally, you can have tags on your objects. So, for example, we could tag base security and add classification equals personal health data information to your objects. So let me show you how that works. So, in this case, let's pretend it's phi data. So what I'm going to do is go to the properties of the subject. Let's click it again and properties. And in here I'm able to add tags. And the tag I'm going to add is classification, and the value is phi for personal health information, and then click on save. And now my object has been tagged with this. And so if I had the right bucket policy or the right Im policy, I could restrict access to this file thanks to this tag, because I've tagged this object to be data. That is quite sensitive. OK, well, that's it. Remember, for security again, bucket policies, encryption, then security, VPC, endpoints, and tags. And that's all you need to know. And I will see you at the next lecture.
Amazon AWS Certified Machine Learning - Specialty Exam Dumps, Amazon AWS Certified Machine Learning - Specialty Practice Test Questions and Answers
Do you have questions about our AWS Certified Machine Learning - Specialty AWS Certified Machine Learning - Specialty (MLS-C01) practice test questions and answers or any of our products? If you are not clear about our Amazon AWS Certified Machine Learning - Specialty exam practice test questions, you can read the FAQ below.
Purchase Amazon AWS Certified Machine Learning - Specialty Exam Training Products Individually
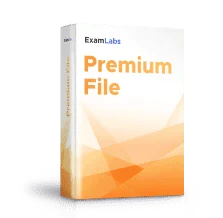
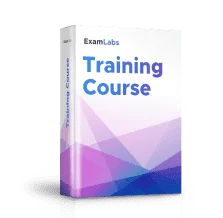
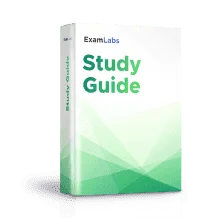
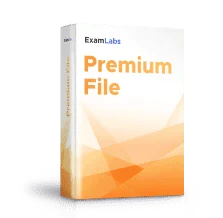
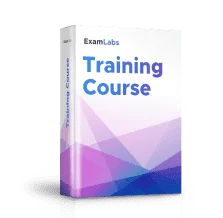
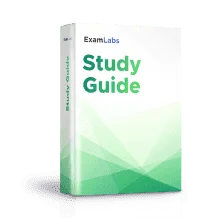