Pass Google Professional Data Engineer Exam in First Attempt Easily
Latest Google Professional Data Engineer Practice Test Questions, Exam Dumps
Accurate & Verified Answers As Experienced in the Actual Test!
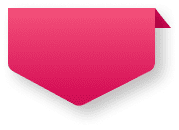
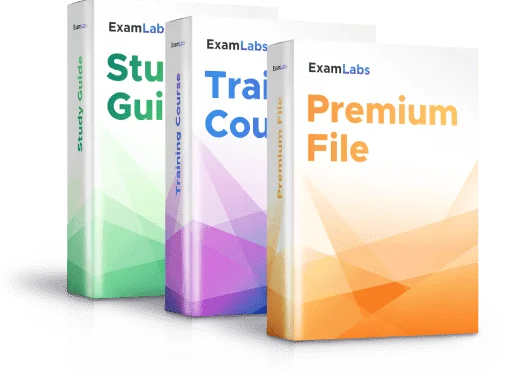
Check our Last Week Results!



- Premium File 319 Questions & Answers
Last Update: Feb 22, 2025 - Training Course 201 Lectures
- Study Guide 543 Pages
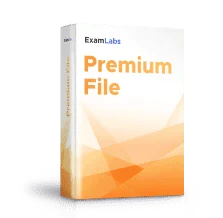
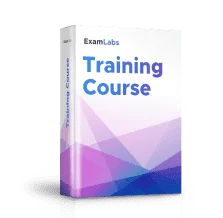
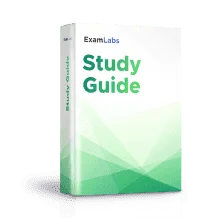
Download Free Google Professional Data Engineer Exam Dumps, Practice Test
File Name | Size | Downloads | |
---|---|---|---|
google |
1.4 MB | 1170 | Download |
google |
2 MB | 1190 | Download |
google |
281.8 KB | 1224 | Download |
google |
406.3 KB | 1329 | Download |
google |
398.7 KB | 1444 | Download |
google |
310.4 KB | 1466 | Download |
google |
317.4 KB | 1984 | Download |
google |
346.3 KB | 2049 | Download |
google |
373.2 KB | 2514 | Download |
Free VCE files for Google Professional Data Engineer certification practice test questions and answers, exam dumps are uploaded by real users who have taken the exam recently. Download the latest Professional Data Engineer Professional Data Engineer on Google Cloud Platform certification exam practice test questions and answers and sign up for free on Exam-Labs.
Comments
Google Professional Data Engineer Practice Test Questions, Google Professional Data Engineer Exam dumps
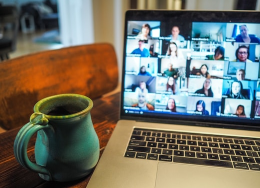
You, This Course and Us
1. You, This Course and Us
Hi, and welcome to this course, The Complete Google Data Engineer and Cloud Architect Guide. We've both been at Google for a long time and have had long experience with these technologies. We've also got to pass the certification exams for Google Data Engineer and Cloud Architect. This course will teach you everything that you need to know to leverage the capabilities of the Google Cloud platform, to pass the certification test, to be a data engineer as well as a cloud architect, and to build TensorFlow machine learning models on the Google Cloud. This course covers a whole range of Google's big data technologies: data flow, DataProc, Big Table, and BigQuery. Along the way, you'll also learn about what compute options are available for app engines, compute engine containers, and Kubernetes. This course also covers machine learning in TensorFlow in a lot of detail and from first principles. It's a very easy and approachable way to learn these technologies. In addition, we'll also cover networking, load balancing, and VPCs, as well as security, OAuth, API keys, service accounts, and identity-aware proxies. You could be an architect looking to move to the cloud, or a young engineer looking for your first introduction to cloud technologies, or a data scientist looking to deploy your TensorFlow machine learning models on the cloud. This course is for you. It's.
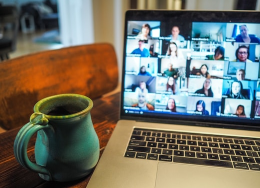
Introduction
1. Theory, Practice and Tests
Hello and welcome to this first module in our course on the Google Cloud Platform. This module will serve as an overview. We will start by introducing the cloud computing paradigm and understand why it's getting so popular these days. One clear and inescapable fact of the world of computing these days is that data sets are growing larger and that in-memory processing on single machines is no longer a viable option. A simple proxy for this is how much data one can fit into a single Excel workbook. And it's clear that there is a lot less that one can accomplish with Excel alone now than maybe ten years ago. Here, distributed computing frameworks such as Hadoop and MapReduce have really helped. Hadoop and MapReduce are standard cookie cutter ways of taking complicated jobs, parallelizing those jobs so that they run on a number of distributed machines, and getting the results back for your convenience. The power and versatility of the Map Reduce programming paradigm and the great design underlying Hadoop have spawned an entire ecosystem of their own. One side effect of this Hadoop ecosystem and its popularity is the rise of clustered or distributed computing. But a simple fact is that configuring a cluster of distributed machines is quite complicated and expensive. Ask yourself: How many companies or organizations do you know that run Hadoop in fully distributed mode with raw Hadoop without making use of a nice company version such as Cloud Era? The answer is probably none. That's because it takes a lot to get Hadoop and MapReduce going in fully distributed mode. And this really is where cloud computing comes into its own. Cloud services such as the Google Cloud Platform, AWS, Amazon's market and industry leader, the Amazon Web Services platform, and Microsoft Azure provide a further level of abstraction. Even on top of the distributed abstraction provided by Hadoop and MapReduce, cloud services have a bunch of advantages. We shall examine those in a little more detail. But in a nutshell, it all really comes down to the need for massively parallel distributed computing, where you as the end user are not responsible for configuring every machine on a large and complex cluster. And that really is what the Google Cloud Platform is all about. And that's what this course is all about as well. Let's also really quickly talk about how this course relates to a couple of things that have to do with the Google Cloud Platform. The first of these is the Google Cloud Data Engineer certification test, and the other is the Google Cloud Architect test. Now, it turns out that we've given and passed both of them, and our advice to you is to not try and prep for the test. I guess what I mean by that is to think of these tests as getting a driver's license. Of course, when you're getting a driving license, your objective is to get the license and start driving. But the other objective that you have is to actually learn how to drive. Clearly, if you have a driving license but don't really know how to drive, you're going to be quite a risk to yourself, your loved ones, and the world at large. So make sure, while preparing for either of these two tests, that you do not prep for the test, because we've seen that lead to some pretty unsatisfactory outcomes. that out of the way. Let's very quickly talk about the tests themselves. The Google Certified Data Engineer test is 2.5 hours 50. Question multiple In our experience, this was not a very easy game to play. That's because the syllabus is pretty broad; it's pretty eclectic, and what's more, it tests even some pretty arcane parts of the syllabus in a lot of detail. I think it's reasonable to say that Cloud Architect is definitely easier. One is more theoretical. It focuses on those parts of the Google Cloud platform, like the compute choices, networking, security, and so on, that are not heavily tested on the Data Engineer test. And so in a sense, these two tests are complementary. The topics that are major or important for the Data Engineer are minor or relatively unimportant for the Cloud Architect, and vice versa. Three broad categories that are really heavily tested, at least in our experience, are big data technologies, storage technologies, and machine learning. Let's really quickly talk about what each of these terms represents. Big data includes BigQuery can be thought of as Google's equivalent to Hive Data Flow, which is a sort of catch-all transformation technology akin to Spark, as well as Pig and Cloud pub sub. This is the way in which one deals with streaming data on the Google Cloud Platform. It's similar to Apache filling or possibly some parts of Spark streaming, i.e. big data. Along the way, collateral will also be tested. A whole bunch of Hadoop ecosystem-related questions HBase or Cassandra, MongoDB, or Redis questions like this show up on the test. And that's why, really, it's no exaggeration to say that a lot of breadth and a lot of time spent working with these technologies is probably the best way to handle big data. Portions of this test, in my opinion, are not very Google specific. The storage technology sections are pretty Google platform specific. Here, what I think will be tested includes cloud storage. More on each of these terms in a minute. Cloud SQL big table. Big Table is Google's equivalent of HBase, which is a key-value index, document, and database. All of these are fairly Google-specific because there are a whole bunch of quirks that are important on the test. And the third section that's really heavily tested on the Data Engineer test has to do with machine learning. Here there are a whole bunch of machine-learning concepts that are tested: supervised and unsupervised learning feature vectors, fitting, stuff like that. Then a whole bunch of TensorFlow related stuff and cloud ML related stuff Now, in our opinion, the machine learning offerings on the Google Cloud Platform are really quite a differentiator. I'm not convinced that big data storage technologies are better or more widely used than competing offerings from Amazon or Microsoft. But TensorFlow, for instance, is clearly the industry-leading framework for deep learning and neural networks. And so that, I think, is why this section of the test is not only pretty important from the test perspective, it's also pretty important from the point of view of getting the most out of the Google Cloud Platform. These are the three major categories of topics. In addition, there are also some minor topics that are covered on the data engineer exam. These are major topics on the Cloud Architect exam. So it's kind of like Yin and Yang. The cloud architect and the data engineer exams are complementary. There is not a whole lot of overlap between them. So again, these topics aren't that important from the data engineer point of view, but they are pretty important from the cloud architect exam point of view. The first has to do with compute choices, the second has to do with operational stuff like logging, monitoring, endpoints, and so on. and lastly, security networking and deployment. The compute choices on the Google Cloud platform are referred to as "App Engine," Compute Engine," and "Container Engine," and we will have a lot more to say about each one of these. Logging and monitoring on the GCP happened via a part of the GCP known as Stackdriver. And then there is a whole bunch of stuff related to security authorization and authentication, API keys, OAuth networking, for instance, load balancing, VPN, and so on. My sense is that if one has good working knowledge of all of this, one can pass both of these certifications, and more importantly, one can be an effective practitioner and an effective architect or data engineer on the Google Cloud platform. Now, let's also talk about the required context. You can think of these as prerequisites. In my opinion, you are going to struggle with these tests and with this content if you're not familiar with Hadoop, Spark, and MapReduce at a fairly detailed and granular level, and that's because these technologies really form the bedrock of data processing, data flow, and really the whole design of the Google Cloud Platform. Then there are technologies like Hive, HBase, and Pig. These are not really important to know from a nitty-gritty point of view. For example, you don't need to understand how bucketing or partitioning work on Hive, but you do need to understand the use cases and some of the implementation quirks of these technologies to get by. For instance, it's important for you to know that HBase is a columnar data store. It's important to know what hot spotting is and how a poor choice of row keys can really adversely affect the performance of your design. And I think it's also pretty important to understand how relational databases work, just the strong fundamentals—how indexing works, how hashing works, what a lookup is, how much time is given to a hash table, how an index is implemented, and so on. Now for a quick word on the drills and labs. This course is structured as an approximately 50-50 mix of drills and labs. And that's on purpose. like we said at the start of this module. There are two use cases for this course. One is if you'd like to clear the Google certifications. The other is if you'd like to be fairly handy around the Google Cloud Platform. From either point of view, the labs and the drills and the implementation stuff are pretty important. On the one hand, the tests do test a little bit of syntax; nothing too hardcore, but it is there. And on the other hand, to do anything at all useful with the GCP, one is going to have to know what the implementations are all about. One last bit of advice on this front, folks. Don't try to prep for the test. These might sound like famous last words, but I honestly believe that these are really the best strategies for getting the most out of this course, the best out of the Google Certification test, and the best out of the Google Club platform. Just kind of go along for the ride, enjoying the process and my senses. At the end of it all, you'll happily pass both of the certification tests.
2. Lab: Setting Up A GCP Account
Let's kickstart this video with a question. You should know the answer to this. When you're done watching this video, try to answer it. It will improve your understanding of the Google Cloud Platform. What is the top-level billing unit in the Google Cloud Platform, which holds all your cloud resources, your virtual machines, your databases, your websites, and anything else that you set up? Try and figure this out while we walk you through setting up a Google Cloud account. This is the first thing that you need to do in order to run all the demos in this course. It's best if you run all your demos using GCP in an incognito window. It will ensure that it will not interfere with the other stuff that you have running on Google. Go to console developers at Google.com and sign in to continue using Google Cloud Platform. If you already have a Gmail account, that's what you'll use to sign in here. If you don't get a Gmail account before you sign in to Google Cloud Platform, if you're doing this for the very first time, it'll take you to a page where it will ask you a bunch of personal information, the country that you belong to, whether you accept all their terms and conditions, and so on. I'm going to assume that you are all newbies here and this is your first time on the Google Cloud Platform. You're familiar with big data technologies, but not with Google Cloud. Here, you get access to all platform products. Google enables a free trial for everyone and gives you $300 of credit for free. So even if you're going to upgrade to a paid account, you won't shell out any money until you reach the $300 limit. Don't worry, all the demos in this course will keep you well within this limit. You won't be out of pocket. Also, if you do happen to use more resources than the $300 limit, Google Cloud will warn you. Before charging your credit card, you can choose to discontinue your free trial at that point in time. Go ahead and take a look at the form that you have to fill out. I don't want to receive any surveys or offers, so I hit no and yes. Yes. I want to agree with all the terms and conditions because I want to use this platform. Hit "Agree" and "Continue" when you're done. This leads us to another form that Google requires us to fill out. You need to provide a credit card number in order to use the free trial. You won't be charged, though, so go and create your payment profile right here on this page. You can choose a business account or an individual account and provide your tax information. It's optional, though, so I haven't filled in the rest of the details. And right there at the very bottom is where you specify your credit card number in order to use the free trial for Google Cloud. I haven't been charged so far, so you can be confident that Google will not unnecessarily take money from your account. Go ahead and fill in your credit card details, and that's it. Your Google Cloud account has been created. You'll be automatically taken to a page, which is the dashboard for your account. And here, the first thing you need to do is to create a new project. This project, which is associated with your Google Cloud account, is the top-level billing instance on the Google Cloud Platform. All your resources belong to a project, and you can specify a different billing account for each project. That way, if you have a bunch of Google Cloud projects going on, you can ask different people to pay for them, maybe different teams within your organization. Every project has a name, which you specify. It is My Test Project in my case, and then ID, which is generated by Google. The project ID contains my project name and a couple of random numbers to make sure that it's unique. The free trial quota for projects is up to eleven projects within your Google Cloud account. We'll be using just one across all our demos. The MyTest project that we just created, I have a business account associated with the Lunacon.com Organization. That's what you see right there on screen. Go ahead, hit Create, and it will spin around a little bit and take some time before your project is set up. Once your project has been created, you'll see a dashboard that looks somewhat like this. There's a lot of stuff going on in here. Let's orient ourselves to this page. At the very top, you can see what project this dashboard is associated with. There's also a drop-down there to allow you to switch between projects easily. The very first card gives us details of the project, such as its name and the associated project ID. There's also a quick link to your project settings page where you can change your billing information and other project-related details. The Compute Engine card shows you a summary of activity on your Compute instances. I have no instances, which is why this card is relatively empty. I get a quick status check on the right indicating that all my services are green. I have no services or resources set up, so that's not surprising. And I can see the billing details of this project at a glance. I haven't been billed anything so far, so that's a relief. These cards are just the highlights, though. You can scroll down to see a lot of other useful information and quick starts from this dashboard. The notifications icon that you can see on the top right gives me platform-wide notifications for all the projects that I own. You can see here the My Test project that we just created, and I have a bunch of older notifications from when I was playing around with Google Cloud Platform earlier. So here we are at the Google Cloud Dashboard. How do we get to all the services that the Cloud Platform makes available to us? You'll use this three-line navigation button at the top left. This is the most important button, and you'll find that during the course of our demo or during the course of using this platform, you'll be navigating here over and over again. Clicking on this will open up the navigation menu, and you'll see all the Cloud Platform services and products available to you just one click away. Take the time to explore this menu. There's a lot of interesting stuff out there, but what we're going to work on first is to create a VM instance, an instance of the Compute Engine. Go to the Compute Engine menu and click on VM instances there. And this will take us to a page where we can create our very first virtual machine instance on Google Cloud. You also now know that all Google Cloud resources, services, or products that you use are built as top-level top level project. You can set up different projects for different teams in your organization.
3. Lab: Using The Cloud Shell
By the end of this video, you'll understand when you should use the Google Cloud Shell command prompt instead of connecting from your local machine to Google Cloud. In this video, I will explore the command line that Google Cloud offers in order to access its resources directly. Open up your Incognito window and access ConsoleCloud.Google.com to get your GCP dashboard, make sure you are signed in, and make sure that my test project is selected. You can also click on the drop-down available there to see what other projects are available. Here you can see my test project. It's the project that we work on. I also have my first project and a project that's associated with my organization. There is some activity on my API dashboard. That's because I just created a VM for practice and that showed up here. Click on the navigation menu with horizontal lines on the top left, and let's look at the billing details for this project. The billing page is something that you need to be familiar with. will be taken to a page that indicates that you're on your free trial and when it expires, and it indicates what projects are linked to this billing account. You must now upgrade to a Premium account in order to access all of the features required for the demos in this course. If you see the "Upgrade" button on the top right of your dashboard, click on it and upgrade to a Premium account. If you don't see it, no worries. Just ignore it and you're fine. Again, you won't be billed unless you exceed your credit limit. All the demos in this course require that you keep billing enabled for this project. Once you're done, you can simply disable billing or delete this project altogether. Before we create our first VM instance, let's understand the cloud share. A Cloud Shell is a machine instance that runs on the Google Cloud, which serves as your command line. All GCP accounts have a Cloud Shell, which they can use to connect to other instances on the cloud platform. The cool thing about the Cloud Shell is that it provides a complete environment for you to connect to various resources in the cloud. The cool thing about it is that you can directly use the G Cloud command line tools to connect the resources in the cloud, create resources, provision them, and so on. You don't need to install or set up anything. The Cloud Shell is what you'd use if, say, your organization does not allow you to download software on your local machine. It's a great alternative in that case, which just works when you first connect to the Cloud Shell or the G Shell. Google has to spin up an active instance to use for this. It might take a little while, so be patient. And there is our Cloud Shell, and you can see that it's associated with this project. The test project It has the Project ID right there on the prompt. Let's take the Gcloud Command Line Tool for a test run. The G Cloud is Google's command line tool, which allows you to work with resources and do a whole bunch of operations. It's especially useful if you want to script operations and just run a script rather than perform it manually over and over again. The G Cloud Auth list will show you all the credentials that can work with my test project. The Cloud Shell is a fully fledged command line in your browser, so you can run Linux commands such as clear and they will just work. Your Google Cloud platform has an associated config, which you can use to set up your default project, the default zone where you want your VM instances set up, and so on. You can view what project is the current default by typing out "Gcloud Config List project." You can see that my test project is the default. If you need help on what commands are available with Gcloud, simply type Gcloud H and you'll see a whole bunch of information. Or if you need help for a particular command, let's say it's the config command, you can simply say "Gcloud ConfigHelp," and this essentially throws up the man page for that particular command. Everything you need is right there on screen. Gcloud Help Config is the same command with a slightly different syntax. The G Cloud Config List command shows you what properties have been set so far on the configuration. This will only display those properties that are different from the defaults. For example, you can see here that the account has been set to [email protected] and the project is my test project ID. To see all config properties settings, including the defaults, you simply say "G Cloud Config List." All the properties that say unset are those that have their default values or are not set at all. Because the Cloud Shell is basically just a command line, you can also change the prompt. You can change how you want the prompt to look by exporting the PS one environment variable, just like you would do on your terminal window on a Mac or Linux system. It's pretty clear that the Cloud Shell is an easy way to get up and running with Google Cloud, and that's what we'll be using in all our demos. You typically use this option when you can't download software to your local machine. If you're going to be intensely developing on the Google Cloud and you can download software, it's better to download the GM Cloud SDK and use that instead. That offers a permanent connection to your instances on the cloud, instead of a temporary VM instance, which has to be spun up in order to use Cloud Shell.
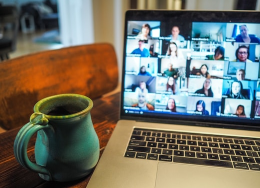
Compute
1. Compute Options
Here's a question that I'd like you to think about. What is the simplest way to host a website on the cloud? So let's say you have a really simple website, just static content and maybe two HTML pages. What's the simplest way for you to get up and running on the Google Cloud Platform? Let's now turn to the second module of this course, which has to do with the compute options available on Google's cloud platform. Compute options are three in number on the cloud platform. The first of these is the app engine, which can be thought of as a platform as a service option. This is serverless and largely ops free. The second compute option is instances of virtual machines, which are known as compute engine virtual instances. These represent the IaaS, or "infra as a service," option. Here, you, as the end user, are responsible for setting up and doing everything that you need. With these virtual machines, you can control every detail of a compute engine instance, right down to the operating system and to whether it has GPUs or not, for instance. In between these two extremes lies the container engine option. Here, you, as the consumer of the cloud platform, set up clusters of machines. These are running a container management service called Kubernetes, and containers are images, in a sense, of your binaries. These are Docker containers. Kubernetes in the Google Cloud Platform will take care of running these. In this case, you have a whole bunch of control, much more than in the app engine world but much less than in the compute engine world. You might find these options ever so slightly abstract. So let's make them real using a case study. Let's see what options you would have if you wanted to set up a website or a web application more generally on the Google Cloud Platform. Let's plunge into this case study. To understand these three options, let's be clear that there are a whole host of cloud use cases. This could include hosting a website, running a Hadoop cluster, or serving a TensorFlow model. TensorFlow is a machine-learning library of neural networks library. The website hosting use case is relatively basic. The Hadoop cluster use case is referred to as a big data use case. It's fairly typical, and the TensorFlow model represents a fairly typical machine learning use case. Across all such cloud use cases, one decision that you as a cloud consumer will have to make is: what compute option do you want to go with? What kind of machines do you want to have running your software? And who will really control those machines? To understand the choices available to us, let's focus in on the simplest of these use cases, the hosting of a website. This is a little world in itself. There is also a spectrum here from the simplest use case, where you have a static website with no SSL, just plain HTML files. All you need is storage space in order to host these files. Maybe your website is a little more sophisticated, so you need some additional bells and whistles. You need, for instance, an SSL layer for checking out. You want to be able to serve rich content, so you need a CDN or a content delivery network. In this case, you are going to need some additional functionality, such as HTTPS serving. You might also want release management. For instance, this is one step up from a static HTML website. This has a bunch of rich content, but it's still relatively static. If you require load balancing or scaling, you must progress to the next level of sophistication. This would happen if you have a sophisticated back end and if you have a large amount of traffic coming into your web app. You now need to make sure that your webapp—and this, by the way, is more of a web app than a website—is smart enough to direct traffic to the appropriate back end instances. Load balancing becomes quite important here. Note also that by definition, if you have different types of back-end instances, that means that you are going to need a greater degree of control over those virtual machines. One step up in sophistication is, if you have a lot of dependencies across those different virtual machines, maybe you've gone with a micro services architecture. In a situation like this, deployment can start to get quite formidable. In recent years, a particular technology that has become very popular for this use case is containers. So here you create a bunch of containers, deploy those containers onto a container cluster, and hope that you have some good way of managing that cluster. At some point along the spectrum, you might find that you are getting into deeper waters than you want to. You might then decide to make use of something like Hiroko or an engine yard. These allow you, in theory, to just focus on the code and leave all of the other complex stuff to the framework. This is a way to retrieve some of the complexity. As the sophistication of your app increases, you might switch to just focusing on the code and give up trying to optimize all of the containers and all of the DevOps stuff. And it turns out that every one of the points on this continuum is nicely encapsulated inside an option available to us under GCP. So let's study all of those options, starting with the simplest. This is the use case where we just have a bunch of static data. No SSL. just plain HTML files. We just need to buy some storage to host these files. Purchasing storage is pretty stupid. Nobody is only buying disk space anymore. At the very least, what you want is auto-scaling, and that's something that's offered to you by GCP. Notice that we still don't have any of the bells and whistles. We don't have HTTPS, CDN, or help with deployment—all of that is still entirely incumbent on us. The option that we go with here is the Google Cloud Storage option. You can think of this as Google's favorite way of allowing you to store relatively immutable, static data such as media or blobs. Hosting on a cloud storage system is pretty simple. You just need to create something called a cloud storage bucket and upload your content to that bucket. GCP will even provide an end point for you. This will be the URL of the form stored at googleapis.com. You, of course, can also use your own domain. Hosting on the cloud is a fairly simple option here. You just write your own HTML or CSS if that's how barebones you'd like to be. You could also use static generators like Jacobor or any of the other options available once you've completed this content. The question is, how do you get it onto cloud storage? Well, you can simply copy it over to your bucket directly. There are a bunch of options here. Remember the web console that we have spoken about? There is also a cloud shell. This is like a command-line utility. Or you could do something a little more sophisticated. You could store it on GitHub and use a web hook to run an update script. Or if you're really feeling your chops, you could even use a CI CD tool like Jenkins and specify some kind of post-build step to push your artifacts to GCP. There is a cloud storage plugin that comes built into Jenkins. Cloud storage is a pretty plain vanilla option, but it is cost-effective and efficient if you just need to serve a bunch of static content or if you mostly work with user-uploaded static media. Note that cloud storage supports a bunch of UGC, or user-generated content. So if your web application requires users to upload video or audio files, or stuff like that, cloud storage could be a perfectly acceptable and very cost-effective alternative for you. This represents the first option that's available to us. This is what we go with. If you have really static data, cloud storage is all that we need. Assume we now require some additional functionality specific to website hosting, such as SSL connectivity, HTTPS serving, or CDN. Here we could make use of some built-in additional functionality in GCP known as "Firebase hosting." Firebase hosting allows us to turn on HTTPS serving. It would allow our end users to tap into CDN edges globally so that they get quick cash access to large content metadata files. Firebase will also offer atomic deployment and one-click rollback. Firebase hosting with Google cloud storage is primarily for storage purposes. This stuff is pretty basic. We've yet to really deploy any code. We aren't really doing anything intelligent in our Web app. That is where the other compute options will come into play. Hopefully all of the territory we've covered has given us enough to answer the question we started this video with. If we really have a simple website, which has static content that rarely changes, to get started and get up and running on the cloud, we just need to get some space in the form of a Google cloud storage bucket. Folks can then access that website either on our domain name or on a Google domain. Something like Storage.Google.com
2. Google Compute Engine (GCE)
Here is a general question that I'd like you to consider. Let's say you have a web application and you're choosing between a SAS, that is, software as a service option, a pass or platform as a service option, something like Hiroko, or an infrastructure service option. Which one of these would you opt for? Remember that fine-grained control over your web app's load balancing is essential here. Which option should you go with? The need for compute options really comes into play once we get to situations involving load balancing or scaling. Here we have a bunch of backend instances doing different types of stuff, different types of computational processing, or maybe accessing a database layer. So now there are clear differences between the operations being carried out by different parts of your web app, and you'd also like to exert more control, for instance, on the load balancing, the scaling, and so on. This is a prototypical IaaS (or infra) use service usecase. What you really want here is a bunch of machines. Those machines are placed at your disposal, and you can go ahead and set them up exactly the way that you would like to. Configuration, administration, management—all of this is now your responsibility. This might sound quite a bit like an on-premise data center or even a colocated setup, but there is one crucial difference: you are absolutely not required to buy the machines yourself. Auto-scaling is taken care of for you. You simply ask for the instances and specify the OS dev stack and the languages. You don't even need to go ahead and install all of these, unless you really need to do some very funky stuff, in which case you probably use containers. In any case, this is the IaaS option, which in Google parlance is Google Compute Engine or GCE. GCE is one of the most standard choices for compute, and this is going to be a workhost topic. You really want to understand it, whether you just want to get the certification or use GCP as an expert; Google Compute Engine still does a lot of hand-holding for you. For instance, there's something called Google Cloud Launcher, which is maybe the easiest way out there to deploy a full web serving stack. You might want to set up something like a lamp or a WordPress application. Cloud Launcher will do that for you in just a few minutes. And what's more, before you deploy all of this, you'll get pretty accurate cost estimates. You'll know roughly how much it's going to cost you to run the site and what versions of the different components you are going to have installed. You can also customize your configuration, for instance, by picking instance names for your virtual machines, choosing machine types, disk sizes, and so on. as we shall see later on when we get to the networking aspects. There are also a whole bunch of cool networking bits that we can accomplish using Google Compute Engine. When you make use of virtual machine instances, you also have some storage options, which you should be aware of. You could go with cloud storage buckets, which we've already discussed. You could also go with standard persistent disks, SSD persistent disks, or local SSDs. These are attached to your individual VM instance. After deployment, you will have full control of your machine instances. As an aside, these storage choices are extremely important. They are very relevant, and we are going to discuss them in excruciating detail in just a little bit. Your virtual machine, obviously, will also run code, and that code can access various storage technologies. Cloud SQL, which has my SQL and PostgresSQL implementations as well as different NoSQL services For instance, each base and hive has its equivalent. Compute Engine also gives you pretty fine-grained control over load balancing. Operations You could carry out load balancing pretty much anywhere in the OSI stack. You could go with a relatively simple network load-balancing version. This operates at level four. You could go with a more complex application, that is, level seven load balancing. We'll discuss load balancing in detail when we get to the networking portions of this class. Do keep in mind a pretty simple rule of thumb: the more sophisticated the load balancing that you would like to do, the higher up in the OSI stack that you need to go. For instance, the most sophisticated load balancing is carried out at the HTTP or HTTPS layer. Here, you can even do stuff like look at cookies for setting session affinity as you go down the OSI stack. As you get to the network layer, the level of sophistication of the load balancing goes down. All of these load balancing operations can be carried out using either GCP's built-in load balancing services or third-party providers. For instance, you might use HTTP load balancing from Njinks, cross-region load balancing from Microsoft IIS, and so on. When you use a computer's engine cluster, you can also carry out internal load balancing, and this can even be auto scaled. For instance, you might want to do this using products such as Ha Proxy and Console. This is a real alphabet soup of third-party tools, and that gets even worse if you're talking about DevOps stuff. If you already have strong in-house DevOps expertise, you can just continue to use all of those tools. For example, you could use management in conjunction with puppet chef salt and ansible. You might automate your image builds with Jenkins Packer Kubernetes, and you might distribute your load testing with Kubernetes. You might have continuous delivery with Travis, and you might have deployments carried out using Spinnaker. There is a reason why I have gone through this long laundry list of DevOps tools, and that's because, as we shall see, there are internal GCP equivalents for a whole bunch of these. You might decide that you do not want to use all of these third-party tools yourself. You want to transfer the owners to the platform. And in that case, all you've got to do is shift to using either containers or App Engine instances. In either one of those options, you'll end up using the Google platform instead. For instance, there is a Cloud Deployment Manager, which you'd use instead of Spinnaker, and a whole bunch of the DevOps stuff would just be abstracted away the moment you switched to containers from Compute Engine instances. Let's come back to the question that we started this video with. The question was, if you would like to exert very fine-grained control over the load balancing of your web app, would you go with a software-as-a-service option, a platform-as-a-service option, or an infra-as-a-service option? So to begin with, this question is ever so slightly ill-formed because it's not quite clear how one could use software as a service for a web app. So let's really eliminate that option as a red herring. That leaves two realistic options: the platform as a service and the infrared service. Now, there are plenty of offerings for hosting websites. Hiroko is one that jumps to mind, but you are not definitely going to get any control over the kind of load balancing that goes on there. So if you really would like to specify specific load balancing types, for instance, content-based load balancing, where all video requests go to specific instances while all other HTML requests go to a different type of instance, then your best option is going with infra as a service on the Google platform. That means that you would opt for hosting using instances of the Google Compute Engine.
Google Professional Data Engineer Exam Dumps, Google Professional Data Engineer Practice Test Questions and Answers
Do you have questions about our Professional Data Engineer Professional Data Engineer on Google Cloud Platform practice test questions and answers or any of our products? If you are not clear about our Google Professional Data Engineer exam practice test questions, you can read the FAQ below.
Purchase Google Professional Data Engineer Exam Training Products Individually
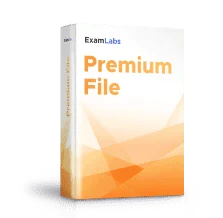
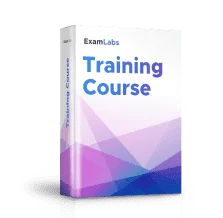
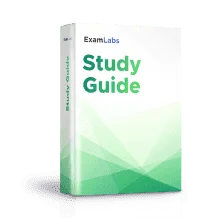
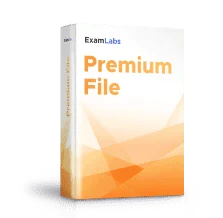
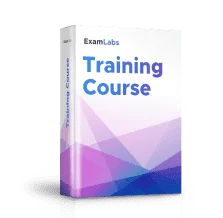
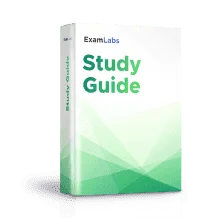
opondo Jnr
Feb 4, 2025, 04:10 PM
Guys, exam-labs has just done it again. these dumps never disappoint. I’m glad to have finally realized my dream of becoming a Google Professional Data Engineer!!!!!!!!!!!! huuuurrraaaaaaaaaaaayyy!!!
khaqan
Jan 26, 2025, 04:07 PM
How helpful is the Professional Data Engineer vce file ...advice ...plz?
saar
Jan 16, 2025, 04:10 PM
The vce file for Professional Data Engineer gave me a real feel of what the actual exam testing environment looks like. I’m anxiously waiting for my exams.
silva_star
Dec 31, 2024, 04:10 PM
wow! I’m now familiar with the exam concepts. can’t wait to take my exam soon. the Professional Data Engineer exam questions from exam-labs online platform have made it possible.
shawnstorm
Dec 19, 2024, 04:09 PM
valid Google Professional Data Engineer exam dumps 4 u here don’t worry.
sasha
Dec 12, 2024, 04:08 PM
I came across the Exam Labs website while making a last ditch effort to find reliable training materials. I tried the Professional Data Engineer practice test and ….it’s what I needed. the questions helped to find my weak areas and I focused on them in my prep process. And…..i passed the exam on my first try))))
juninho
Nov 30, 2024, 04:08 PM
I feel refreshed and ready to take my certification exams. this is after going through Professional Data Engineer questions and answers from the exam-labs website that are designed in the form of the real exam question format. all the exam topics were well covered as per the exam domains.
williams
Nov 20, 2024, 04:08 PM
passing the IT certification exams has always been a challenge to me but for the first time I could smile while coming out of the venue. thank you exam-labs for providing fully updated Google Professional Data Engineer dumps for passing this exam hassle free.